Understanding Bayesian Spam Filters: The Key to Effective Email Management
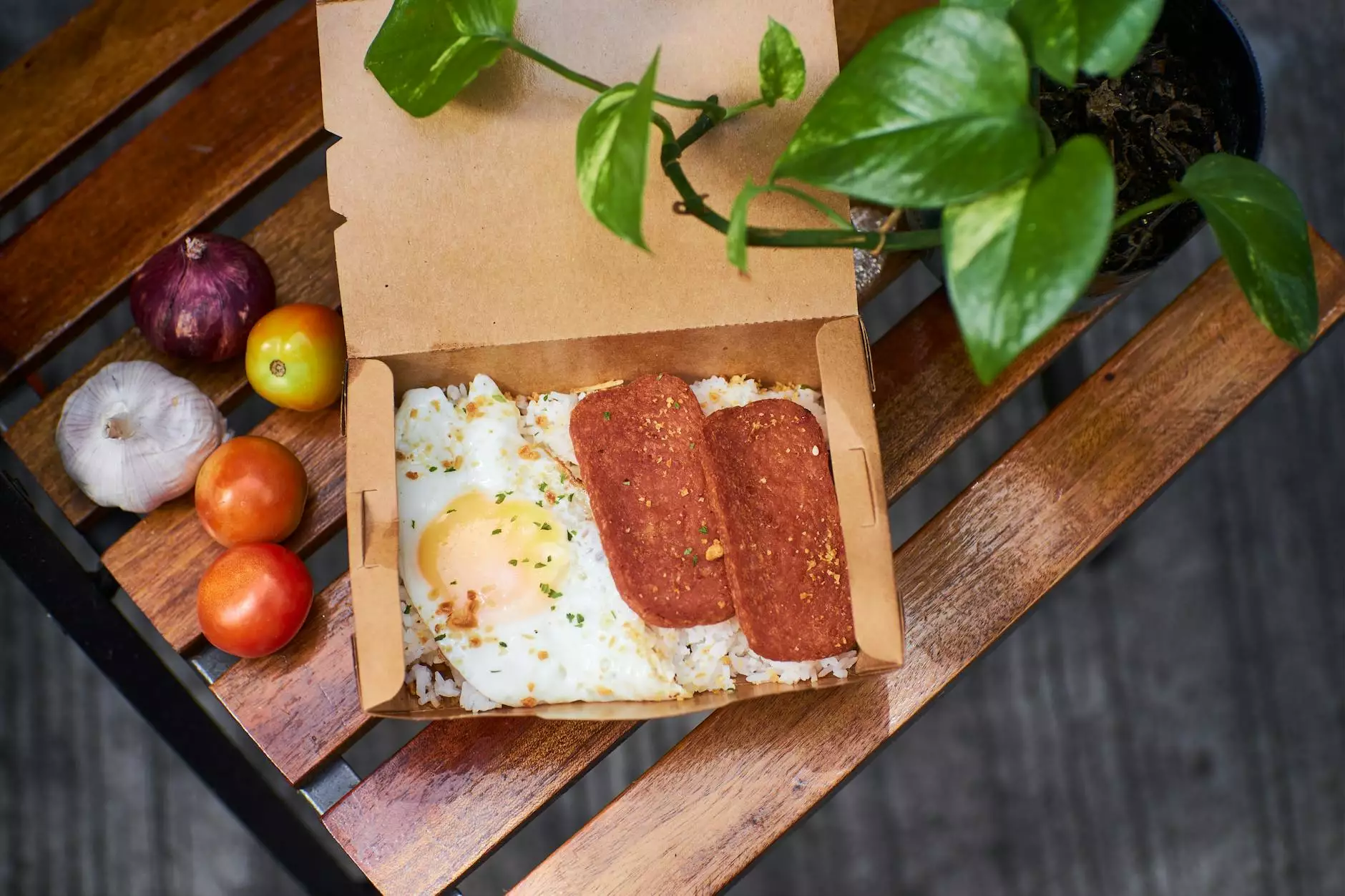
In today's digital landscape, *communication* is predominantly facilitated through email. However, with the advantages of instant messaging comes the significant downside of unwanted correspondence, commonly known as *spam*. Spam can overwhelm inboxes, hinder productivity, and pose cybersecurity threats. This is where effective filtering systems come into play, notably the Bayesian spam filter, which utilizes advanced algorithms to identify and categorize email messages. In this article, we will delve into the workings of Bayesian spam filters, their vital role within IT services and computer repair, and their importance in security systems.
What Is a Bayesian Spam Filter?
A Bayesian spam filter is a statistical method used to classify emails as either *spam* or *ham* (non-spam). The core of this technology is based on Bayes' Theorem, a principle from statistical probability which provides a method for adjusting the probability of a certain event based on prior knowledge. By analyzing the frequency of words and phrases in known spam and non-spam emails, the filter builds a model that predicts the likelihood of incoming messages being spam.
Bayes' Theorem Explained
To comprehend how a Bayesian spam filter operates, one must first understand Bayes' Theorem. This theorem can be expressed mathematically as:
P(A|B) = (P(B|A) * P(A)) / P(B)
In this formula:
- P(A|B) is the probability of event A occurring given that B is true.
- P(B|A) is the probability of event B occurring given that A is true.
- P(A) and P(B) are the probabilities of events A and B occurring independently.
The Mechanism of Bayesian Spam Filtering
The mechanism behind the Bayesian spam filter involves several key steps:
1. Training the Filter
The efficiency of a Bayesian spam filter relies heavily on its training phase. Users provide the filter with a corpus of emails categorized into spam and ham. This dataset allows the filter to analyze common words and phrases in both categories, helping to establish a baseline for classification.
2. Analyzing Incoming Emails
When a new email arrives, the filter breaks down the email's content and assesses the frequency of particular words or phrases. It then calculates the probability of the email being spam based on the model created during the training phase.
3. Making a Decision
If the probability of the email being spam exceeds a predetermined threshold, the filter categorizes it as spam. If it falls below that threshold, it's classified as ham. This dynamic allows the filter to adapt to new spam trends over time.
Benefits of Bayesian Spam Filters for Businesses
Implementing a Bayesian spam filter offers numerous advantages for organizations:
- Increased Productivity: By reducing the influx of unwanted emails, employees can focus on their tasks without distraction.
- Enhanced Security: Bayesian filters help mitigate the risk of phishing attacks and malicious spam, protecting sensitive business data.
- Customizable Thresholds: Businesses can adjust spam detection thresholds to suit their specific communication needs.
- Continuous Improvement: The filter evolves with changing spam tactics, ensuring ongoing effectiveness.
- Cost-Effective: Compared to other spam management solutions, Bayesian spam filters are often part of broader email services, providing value without significant additional cost.
Implementing Bayesian Spam Filters in IT Services
For businesses offering IT services and computer repair, integrating Bayesian spam filters can greatly enhance service offerings. Here's how:
1. IT Support Enhancement
IT service providers can offer email management as part of their support services. By implementing Bayesian spam filters, they can assist clients in keeping their inboxes clean, thus improving overall satisfaction.
2. Proactive Threat Management
IT professionals can monitor and fine-tune spam filters, analyzing the effectiveness of the filter and updating training data periodically to adapt to evolving spam tactics.
3. Training and Awareness
Part of providing IT services involves educating clients on the nature of spam and phishing threats, empowering them with knowledge on how Bayesian filters work and the importance of email hygiene.
The Role of Bayesian Spam Filters in Security Systems
In today’s cybersecurity landscape, the need for advanced security systems is paramount. Here’s the significance of Bayesian spam filters in that realm:
1. Phishing Detection and Prevention
Phishing attacks are one of the most common vectors for cybercrime. By accurately identifying and filtering spam emails, organizations significantly reduce their exposure to phishing attempts.
2. Reducing Malware Risks
Spammers often distribute malware through email attachments. Bayesian spam filters help intercept these malicious emails before they reach the user's inbox, providing an additional layer of defense.
3. Compliance and Data Protections
Many industries are governed by regulations that mandate data protection. By effectively managing email communications and reducing spam, businesses demonstrate compliance and protect sensitive information.
Challenges and Limitations of Bayesian Spam Filters
While Bayesian spam filters are powerful tools, they have limitations that organizations should consider:
1. False Positives
There is a risk of legitimate emails being incorrectly identified as spam, which could result in miscommunication within a business.
2. Requirement of Quality Training Data
The accuracy of the filter is contingent upon the quality and quantity of training data. Inconsistent or poorly categorized data could hinder effectiveness.
3. Maintenance Needs
In order to maintain optimal performance, regular updates and training is necessary to adapt to new spam strategies.
Best Practices for Utilizing Bayesian Spam Filters
To maximize the effectiveness of Bayesian spam filters, consider the following best practices:
1. Regular Training
Continuously train the filter with new and emerging spam samples to keep up with changing patterns.
2. User Education
Educate employees on recognizing spam and the importance of marking emails correctly to improve filter learning.
3. Monitor Filter Performance
Utilize analytics to monitor the filter's performance, looking for patterns in false positives and negatives to adjust settings as needed.
Conclusion
The Bayesian spam filter represents a robust solution to a pervasive problem in the digital communication era. By leveraging statistical methodologies, businesses can gain control over their email communications, enhancing productivity and security in their operations. As organizations like *Spambrella* offer IT services and computer repair, they should consider incorporating Bayesian filters into their offerings. The ability to efficiently manage spam not only protects against threats but also fosters a more effective working environment.