Revolutionizing Image Labeling with Automatic Image Annotation Tools
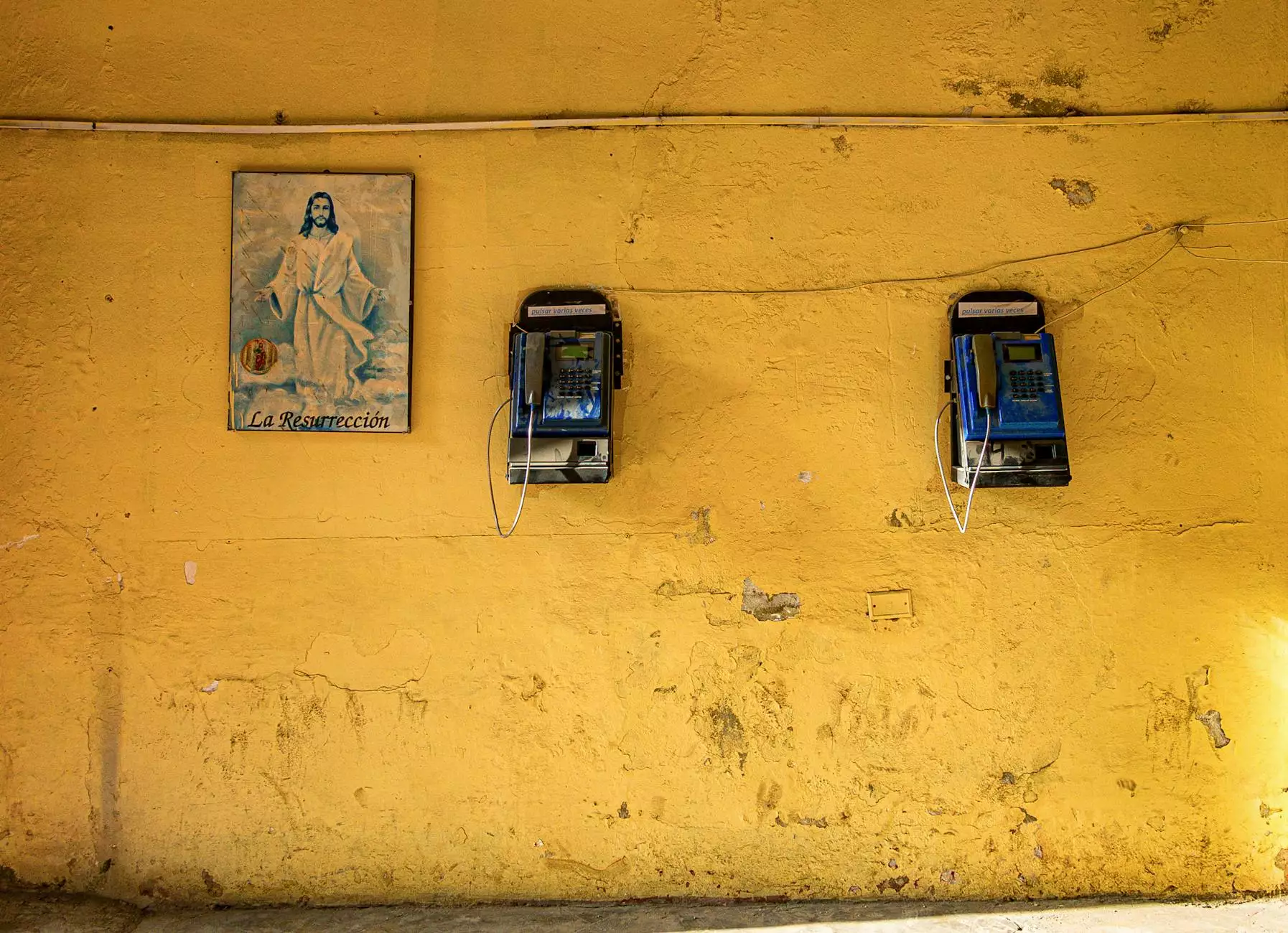
The rapid advancement of technology has brought about unprecedented changes in the way businesses operate, particularly in the realm of data annotation. A critical component for the development of machine learning and artificial intelligence models, effective data annotation is paramount. One of the most significant innovations in this field is the automatic image annotation tool, which streamlines and enhances the image labeling process.
Understanding the Need for Automatic Image Annotation Tools
In our data-driven world, businesses continuously seek ways to improve efficiency and accuracy. Manual image annotation is not only time-consuming but can also introduce human error, leading to discrepancies that can affect the performance of AI models. Here’s why automatic image annotation tools are becoming indispensable:
- Speed: These tools significantly reduce the time required to label images compared to manual processes.
- Consistency: Automated tools ensure uniformity in labeling, maintaining a high standard across all data sets.
- Scalability: Businesses can handle large volumes of data without compromising on quality.
- Cost-Efficiency: Reducing the reliance on human labor for repetitive tasks cuts operational costs.
How Automatic Image Annotation Works
At its core, an automatic image annotation tool utilizes machine learning algorithms to identify and label objects within images. Here's a breakdown of how this sophisticated technology operates:
1. Data Collection
The first step is gathering a substantial repository of labeled images. This serves as the training data that feeds the machine learning model.
2. Training the Model
Using techniques like supervised learning, the model is trained to recognize patterns and features in the images. The more diverse and extensive the training data, the better the model learns to generalize from one image to another.
3. Image Annotation
Once trained, the model can quickly analyze new images, identifying and labeling objects autonomously. This label generation occurs through various methods, including bounding boxes, segmentation masks, or keypoints.
4. Quality Assurance
Although these tools are automated, it’s crucial to have a quality assurance phase where human annotators review the labels to ensure they meet the required standards.
Benefits of Using an Automatic Image Annotation Tool
When businesses integrate an automatic image annotation tool into their data processing workflow, they unlock a host of benefits:
Enhanced Productivity
By automating the tedious process of image labeling, teams can focus on more strategic tasks, thus boosting overall productivity.
Better Resource Allocation
Companies can reallocate human resources that were previously tied up in manual annotation to areas where creative and critical thinking are needed.
Improved Accuracy Rates
The precision offered by machine learning models helps minimize errors often seen in manual annotations, increasing the reliability of the data used for AI training.
Accelerated Time-to-Market
For businesses looking to develop and deploy machine learning applications rapidly, swift data preparation facilitated by automatic annotation tools enables a faster time-to-market.
Key Features of an Effective Automatic Image Annotation Tool
When choosing an automatic image annotation tool, it's vital to consider features that enhance usability and effectiveness. Here are some key features to look for:
- Real-Time Annotation: The ability to annotate images in real-time allows teams to work collaboratively and increase productivity.
- Support for Multiple Formats: A versatile tool should support various image formats, ensuring compatibility with existing datasets.
- Advanced Segmentation: Look for tools that offer semantic and instance segmentation capabilities to improve the granularity of annotations.
- User-Friendly Interface: A straightforward interface helps reduce the learning curve and allows users to maximize the tool’s potential effortlessly.
- Integration Capabilities: The ability to integrate with existing workflow systems such as labeling platforms or machine learning pipelines is essential for seamless operations.
Comparing Different Types of Automatic Image Annotation Tools
The market offers various automatic image annotation tools, each with its strengths and weaknesses. Here’s a comparison of some common types:
1. Cloud-Based Tools
Cloud-based automatic annotation tools offer flexibility and accessibility. They allow for collaboration across teams and ensure that all users have access to the latest features and updates.
2. On-Premises Tools
On-premises solutions provide businesses with more control over their data. This can be desirable for companies dealing with sensitive information.
3. Open Source Tools
Open-source image annotation tools enable organizations to customize the software to meet their specific requirements but may require more in-house expertise for deployment and maintenance.
4. Enterprise Solutions
These offerings often come with comprehensive support and advanced features, ideal for large organizations with extensive datasets and complex needs.
Choosing the Right Automatic Image Annotation Tool for Your Business
Selecting the right automatic image annotation tool involves considering several factors tailored to your organization's needs:
- Define Your Requirements: Analyze your annotation requirements based on the complexity and scale of your projects.
- Evaluate Scalability: Ensure the tool can grow with your business needs—whether that’s handling larger datasets or incorporating additional features later.
- Assess Integration: Determine how well the tool will fit into your existing infrastructure and workflows.
- Consider Support and Training: Look for vendors offering robust customer support and training resources to help your team effectively use the software.
- Read Reviews and Testimonials: Gain insights from other users regarding their experiences and outcomes when using the tool.
The Future of Automatic Image Annotation
The landscape of data annotation is continuously evolving, and the future of automatic image annotation tools is poised for rapid advancement. Emerging technologies like deep learning and neural networks are already paving the way for enhanced automation capabilities. Here are some trends to watch in the future:
1. Enhanced AI Capabilities
As artificial intelligence continues to evolve, we can expect automatic annotation tools to deliver even higher accuracy and efficiency. Improving algorithms will lead to better understanding and recognition of complex images.
2. Greater Customization
Tools will likely offer more customization options, allowing businesses to tailor the annotation process to fit specific project requirements.
3. Expanded Use Cases
The applications for automatic image annotation will extend beyond traditional fields, penetrating areas such as healthcare, agriculture, and autonomous vehicles to name a few.
4. Collaborative Annotation Environments
Future tools may provide enhanced collaboration features, enabling multiple teams to annotate together in a more integrated manner, thus speeding up project completion.
Conclusion: The Imperative of Automatic Image Annotation in Modern Business
The integration of an automatic image annotation tool is not just a trend; it is a necessity for businesses aiming to harness the power of machine learning and artificial intelligence effectively. By streamlining image labeling processes, enhancing accuracy, and significantly reducing time and costs, these tools are set to become a cornerstone in the data annotation landscape.
As organizations look towards a future driven by data, investing in the right automatic image annotation tool will be critical for maintaining a competitive edge. To delve deeper into the capabilities of image annotation tools, consider exploring the offerings at KeyLabs AI, where innovation meets efficiency.